#Explainable AI (XAI)
Explore tagged Tumblr posts
Text
What is Explainable AI (XAI): Importance and Use Cases
Explainable AI has been among the most critical developments in this fast-changing revolution of Artificial Intelligence.
Read: https://www.aspiresoftserv.com/blog/guide-on-explainable-ai?utm_source=pin&utm_medium=dk&utm_campaign=link
0 notes
Text
AI Trading
What is AI and Its Relevance in Modern Trading? 1. Definition of AI Artificial Intelligence (AI): A branch of computer science focused on creating systems capable of performing tasks that typically require human intelligence. These tasks include learning, reasoning, problem-solving, understanding natural language, and perception. Machine Learning (ML): A subset of AI that involves the…
#AI and Market Sentiment#AI and Market Trends#AI in Cryptocurrency Markets#AI in Equity Trading#AI in Finance#AI in Forex Markets#AI Trading Strategies#AI-Driven Investment Strategies#AI-Powered Trading Tools#Artificial Intelligence (AI)#Automated Trading Systems#Backtesting Trading Models#Blockchain Technology#Crypto Market Analysis#cryptocurrency trading#Data Quality in Trading#Deep Learning (DL)#equity markets#Event-Driven Trading#Explainable AI (XAI)#Financial Markets#forex trading#Human-AI Collaboration#learn technical analysis#Machine Learning (ML)#Market Volatility#Natural Language Processing (NLP)#Portfolio Optimization#Predictive Analytics in Trading#Predictive Modeling
0 notes
Text
Can AI Explain Itself? Unveiling the Mystery Behind Machine Decisions with a Data Science Course
Artificial intelligence has become ubiquitous in our lives, from influencing our social media feeds to powering self-driving cars. However, the inner workings of many AI models remain shrouded in mystery. This lack of transparency, often referred to as the "black box" problem, raises critical questions: How are these decisions made? Can we trust AI to make fair and unbiased choices?
This is where Explainable AI (XAI) comes in. XAI aims to shed light on the decision-making processes of AI models, allowing us to understand why a particular prediction was made or a specific recommendation was offered. A well-designed data science course can equip you with the knowledge and skills to navigate the world of XAI and contribute to the development of more transparent and trustworthy AI systems.
Unveiling the Black Box: Why Explainability Matters in AI
The lack of explainability in AI raises several concerns:
Bias and Fairness: AI models can perpetuate societal biases present in the data they are trained on. Without understanding how these models arrive at their decisions, it's difficult to identify and mitigate potential bias.
Accountability and Trust: When an AI system makes a critical decision, such as denying a loan application or flagging someone for security reasons, it's crucial to explain the rationale behind the decision. This fosters trust and accountability in AI systems.
Debugging and Improvement: If an AI model consistently makes inaccurate predictions, being able to explain its reasoning is essential for debugging and improving its performance.
XAI offers various techniques to make AI models more interpretable. Here are a few examples:
Feature Importance: This technique identifies the input features that have the most significant influence on the model's output. By understanding which features matter most, we gain insights into the model's decision-making process.
Decision Trees: Decision trees represent the model's logic in a tree-like structure, where each branch represents a decision point based on specific features. This allows for a clear visualization of the steps leading to the final prediction.
LIME (Local Interpretable Model-Agnostic Explanations): LIME generates local explanations for individual predictions, providing insights into why a specific instance received a particular outcome.
Unlocking the Power of XAI: What a Data Science Course Offers
A comprehensive data science course plays a crucial role in understanding and applying XAI techniques. Here's what you can expect to gain:
Foundational Knowledge: The program will provide a solid foundation in machine learning algorithms, the very building blocks of AI models. Understanding these algorithms forms the basis for understanding how they make predictions.
Introduction to XAI Techniques: The course will delve into various XAI methodologies, equipping you with the ability to choose the most appropriate technique for a specific AI model and application.
Hands-on Learning: Through practical projects, you'll gain experience applying XAI techniques to real-world datasets. This hands-on approach solidifies your understanding and allows you to experiment and explore different XAI approaches.
Ethical Considerations: A data science course that incorporates XAI will also address the ethical considerations surrounding AI development and deployment. You'll learn how XAI can be used to mitigate bias and ensure fairness in AI systems.
Beyond technical skills, a data science course fosters critical thinking, problem-solving abilities, and the capacity to communicate complex information effectively. These skills are essential for success in the field of XAI, where clear communication of technical concepts to stakeholders is crucial.
The Future of AI: Transparency and Trust
As AI continues to evolve and integrate further into our lives, XAI plays a vital role in building trust and ensuring responsible AI development. By fostering transparency and explainability, XAI empowers us to understand how AI systems work, identify potential biases, and ultimately, hold these systems accountable.
A data science course equips you with the necessary tools and knowledge to become a key player in this critical field. Whether you're interested in developing explainable AI models, interpreting their outputs, or advocating for ethical AI practices, a data science course can pave the way for a rewarding career at the forefront of this transformative technology.
If you're passionate about artificial intelligence and want to contribute to a future where AI decisions are transparent and trustworthy, then consider enrolling in a well-designed data science course. It can be the first step on your journey to demystifying the black box of AI and unlocking the true potential of this powerful technology.
0 notes
Text
Explaining explainable artificial intelligence (XAI)
What is explainable AI and why is it critical for high-stakes artificial intelligence applications? #XAI #AI #high-stakes
Explainable AI (XAI) is a subfield of Artificial Intelligence (AI) that makes machine learning models more transparent and interpretable to humans. Explainable AI helps clarify how AI figures out specific solutions, like classification or spotting objects. It can also answer basic (wh) questions, shedding light on the why and how behind AI decisions. This explainability, which is not possible in…
View On WordPress
0 notes
Text
Exploring Explainable AI (XAI) and its Benefits for AI Systems
Hey everyone! I'm excited to share my latest blog post on Explainable AI. Check it out on my website now and let me know your thoughts in the comments. #ai #technews #xai
Artificial Intelligence (AI) has become a ubiquitous presence in our lives, from recommending products on e-commerce sites to powering the latest autonomous vehicles. However, as AI becomes more prevalent, there is a growing concern about its accountability and transparency. That’s where Explainable AI (XAI) comes into play. In this blog post, we’ll explore what Explainable AI is and its…
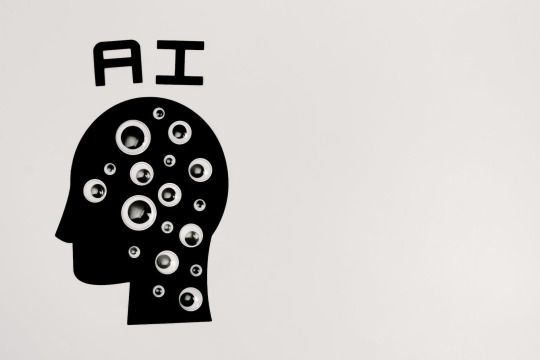
View On WordPress
#AI#artificial intelligence#Bias#Explainable AI#Google Cloud AutoML Vision#IBM Explainable AI Toolkit#machine learning#Transparency#Trustworthiness#XAI
1 note
·
View note
Text
ADDRESSING TWITTER'S TOS/POLICY IN REGARDS TO ARTISTS AND AI
Hi !! if you're an artist and have been on twitter, you've most likely seen these screen shots of twitters terms of service and privacy policy regarding AI and how twitter can use your content
I want to break down the information that's been going around as I noticed a lot of it is unintentionally misinformation/fearmongering that may be causing artists more harm than good by causing them to panic and leave the platform early
As someone who is an artist and makes a good amount of my income off of art, I understand the threat of AI art and know how scary it is and I hope to dispel some of this fear regarding twitter's TOS/Privacy policy at least. At a surface level yes, what's going on seems scary but there's far more to it and I'd like to explain it in more detail so people can properly make decisions!
This is a long post just as a warning and all screenshots should have an alt - ID with the text and general summary of the image
Terms of Service
Firstly, lets look at the viral post regarding twitter's terms of service and are shown below
I have seen these spread a lot and have seen so many people leave twitter/delete all their art/deactivate there when this is just industry standard to include in TOS
Below are other sites TOS I found real quick with the same/similar clauses! From instagram, tiktok, and even Tumblr itself respectively, with the bit worded similar highlighted
Even Bluesky, a sight viewed as a safe haven from AI content has this section
As you can see, all of them say essentially the same thing, as it is industry standard and it's necessary for sites that allow you to publish and others to interact with your content to prevent companies from getting into legal trouble.
Let me break down some of the most common terms and how these app do these things with your art/content:
storing data - > allowing you to keep content uploaded/stored on their servers (Ex. comments, info about user like pfp)
publishing -> allowing you to post content
redistributing -> allowing others to share content, sharing on other sites (Ex. a Tumblr post on twitter)
modifying -> automatic cropping, in app editing, dropping quality in order to post, etc.
creating derivative works -> reblogs with comments, quote retweets where people add stuff to your work, tiktok stitches/duets
While these terms may seems intimidating, they are basically just tech jargon for the specific terms we know used for legal purposes, once more, simply industry standard :)
Saying that Twitter "published stored modified and then created a derivative work of my data without compensating me" sounds way more horrible than saying "I posted my art to twitter which killed the quality and cropped it funny and my friend quote-tweeted it with 'haha L' " and yet they're the same !
Privacy Policy
This part is more messy than the first and may be more of a cause for concern for artists. It is in regards to this screenshot I've seen going around
Firstly, I want to say that that is the only section in twitter's privacy policy where AI /machine learning is mentioned and the section it is is regarding how twitter uses user information.
Secondly, I do want to want to acknowledge that Elon Musk does have an AI development company, xAI. This company works in the development of AI, however, they want to make a good AGI which stands for artificial general intelligence (chatgpt, for example, is another AGI) in order to "understand the universe" with a scientific focus. Elon has mentioned wanting it to be able to solve complex mathematics and technical problems. He also, ofc, wants it to be marketable. You can read more about that here: xAI's website
Elon Musk has claimed that xAI will use tweets to help train it/improve it. As far as I'm aware, this isn't happening yet. xAI also, despite the name, does NOT belong/isn't a service of Xcorp (aka twitter). Therefore, xAI is not an official X product or service like the privacy policy is covering. I believe that the TOS/the privacy policies would need to expand to disclaim that your information will be shared specifically with affiliates in the context of training artificial intelligence models for xAI to be able to use it but I'm no lawyer. (also,,,Elon Musk has said cis/cisgender is a slur and said he was going to remove the block feature which he legally couldn't do. I'd be weary about anything he says)
Anyway, back to the screenshot provided, I know at a glance the red underlined text where it says it uses information collected to train AI but let's look at that in context. Firstly, it starts by saying it uses data it collects to provide and operate X products and services and also uses this data to help improve products to improve user's experiences on X and that AI may be used for "the purposes outlined in this policy". This means essentially just that is uses data it collects on you not only as a basis for X products and services (ex. targeting ads) but also as a way for them to improve (ex. AI algorithms to improve targeting ads). Other services it lists are recommending topics, recommending people to follow, offering third-party services, allowing affiliates etc. I believe this is all the policy allows AI to be used for atm.
An example of this is if I were to post an image of a dog, an AI may see and recognize the dog in my image and then suggest me more dog content! It may also use this picture of a dog to add to its database of dogs, specific breeds, animals with fur, etc. to improve this recommendation feature.
This type of AI image, once more, is common in a lot of media sites such as Tumblr, insta, and tiktok, and is often used for content moderation as shown below once more
Again, as far as I'm aware, this type of machine learning is to improve/streamline twitter's recommendation algorithm and not to produce generative content as that would need to be disclaimed!!
Claiming that twitter is now using your art to train AI models therefore is somewhat misleading as yes, it is technically doing that, as it does scan the images you post including art. However, it is NOT doing it to learn how to draw/generate new content but to scan and recognize objects/settings/etc better so it can do what social media does best, push more products to you and earn more money.
(also as a small tangent/personal opinion, AI art cannot be copywritten and therefore selling it would be a very messy area, so I do not think a company driven by profit and greed would invest so much in such a legally grey area)
Machine learning is a vast field , encompassing WAY More than just art. Please don't jump to assume just because AI is mentioned in a privacy policy that that means twitter is training a generative AI when everything else points to it being used for content moderation and profit like every other site uses it
Given how untrustworthy and just plain horrible Elon Musk is, it is VERY likely that one day twitter and xAI will use user's content to develop/train a generative AI that may have an art aspect aside from the science focus but for now it is just scanning your images- all of them- art or not- for recognizable content to sell for you and to improve that algorithm to better recognize stuff, the same way Tumblr does that but to detect if there's any nsfw elements in images.
WHAT TO DO AS AN ARTIST?
Everyone has a right to their own opinion of course ! Even just knowing websites collect and store this type of data on you is a valid reason to leave and everyone has their own right to leave any website should they get uncomfortable !
However, when people lie about what the TOS/privacy policy actually says and means and actively spread fear and discourage artists from using twitter, they're unintentionally only making things worse for artists with no where to go.
Yes twitter sucks but the sad reality is that it's the only option a lot of artists have and forcing them away from that for something that isn't even happening yet can be incredibly harmful, especially since there's not really a good replacement site for it yet that isn't also using AI / has that same TOS clause (despite it being harmless)
I do believe that one day xAI will being using your data and while I don't think it'll ever focus solely on art generation as it's largely science based, it is still something to be weary of and it's very valid if artists leave twitter because of that! Yet it should be up to artists to decide when they want to leave/deactivate and I think they should know as much information as possibly before making that decision.
There's also many ways you can protect your art from AI such as glazing it, heavily watermarking it, posting links to external sites, etc. Elon has also stated he'll only be using public tweets which means privating your account/anything sent in DMS should be fine!!
Overall, I just think if we as artists want any chance of fighting back against AI we have to stay vocal and actively fight against those who are pushing it and abandon and scatter at the first sign of ANY machine learning on websites we use, whether it's producing generative art content or not.
Finally, want to end this by saying that this is all just what I've researched by myself and in some cases conclusions I've made based on what makes the most sense to me. In other words, A Lot Could Be Wrong ! so please take this with a grain of salt, especially that second part ! Im not at all any AI/twitter expert but I know that a lot of what people were saying wasn't entirely correct either and wanted to speak up ! If you have anything to add or correct please feel free !!
28 notes
·
View notes
Text
Building Ethical AI: Challenges and Solutions
Artificial Intelligence (AI) is transforming industries worldwide, creating opportunities for innovation, efficiency, and growth. According to recent statistics, the global AI market is expected to grow from $59.67 billion in 2021 to $422.37 billion by 2028, at a CAGR of 39.4% during the forecast period. Despite the tremendous potential, developing AI technologies comes with significant ethical challenges. Ensuring that AI systems are designed and implemented ethically is crucial to maximizing their benefits while minimizing risks. This article explores the challenges in building ethical AI and offers solutions to address these issues effectively.
Understanding Ethical AI
Ethical AI refers to the development and deployment of AI systems in a manner that aligns with widely accepted moral principles and societal values. It encompasses several aspects, including fairness, transparency, accountability, privacy, and security. Ethical AI aims to prevent harm and ensure that AI technologies are used to benefit society as a whole.
The Importance of Ethical AI
Trust and Adoption: Ethical AI builds trust among users and stakeholders, encouraging widespread adoption.
Legal Compliance: Adhering to ethical guidelines helps companies comply with regulations and avoid legal repercussions.
Social Responsibility: Developing ethical AI reflects a commitment to social responsibility and the well-being of society.
Challenges in Building Ethical AI
1. Bias and Fairness
AI systems can inadvertently perpetuate or even amplify existing biases present in the training data. This can lead to unfair treatment of individuals based on race, gender, age, or other attributes.
Solutions:
Diverse Data Sets: Use diverse and representative data sets to train AI models.
Bias Detection Tools: Implement tools and techniques to detect and mitigate biases in AI systems.
Regular Audits: Conduct regular audits to ensure AI systems remain fair and unbiased.
2. Transparency and Explainability
AI systems, especially those based on deep learning, can be complex and opaque, making it difficult to understand their decision-making processes.
Solutions:
Explainable AI (XAI): Develop and use explainable AI models that provide clear and understandable insights into how decisions are made.
Documentation: Maintain thorough documentation of AI models, including data sources, algorithms, and decision-making criteria.
User Education: Educate users and stakeholders about how AI systems work and the rationale behind their decisions.
3. Accountability
Determining accountability for AI-driven decisions can be challenging, particularly when multiple entities are involved in developing and deploying AI systems.
Solutions:
Clear Governance: Establish clear governance structures that define roles and responsibilities for AI development and deployment.
Ethical Guidelines: Develop and enforce ethical guidelines and standards for AI development.
Third-Party Audits: Engage third-party auditors to review and assess the ethical compliance of AI systems.
4. Privacy and Security
AI systems often rely on vast amounts of data, raising concerns about privacy and data security.
Solutions:
Data Anonymization: Use data anonymization techniques to protect individual privacy.
Robust Security Measures: Implement robust security measures to safeguard data and AI systems from breaches and attacks.
Consent Management: Ensure that data collection and use comply with consent requirements and privacy regulations.
5. Ethical Design and Implementation
The design and implementation of AI systems should align with ethical principles from the outset, rather than being an afterthought.
Solutions:
Ethical by Design: Incorporate ethical considerations into the design and development process from the beginning.
Interdisciplinary Teams: Form interdisciplinary teams that include ethicists, sociologists, and other experts to guide ethical AI development.
Continuous Monitoring: Continuously monitor AI systems to ensure they adhere to ethical guidelines throughout their lifecycle.
AI Development Companies and Ethical AI
AI development companies play a crucial role in promoting ethical AI. By adopting ethical practices, these companies can lead the way in creating AI technologies that benefit society. Here are some key steps that AI development companies can take to build ethical AI:
Promoting Ethical Culture
Leadership Commitment: Ensure that leadership is committed to ethical AI and sets a positive example for the entire organization.
Employee Training: Provide training on ethical AI practices and the importance of ethical considerations in AI development.
Engaging with Stakeholders
Stakeholder Involvement: Involve stakeholders, including users, in the AI development process to gather diverse perspectives and address ethical concerns.
Feedback Mechanisms: Establish mechanisms for stakeholders to provide feedback and report ethical concerns.
Adopting Ethical Standards
Industry Standards: Adopt and adhere to industry standards and best practices for ethical AI development.
Collaborative Efforts: Collaborate with other organizations, research institutions, and regulatory bodies to advance ethical AI standards and practices.
Conclusion
Building ethical AI is essential for ensuring that AI technologies are used responsibly and for the benefit of society. The challenges in creating ethical AI are significant, but they can be addressed through concerted efforts and collaboration. By focusing on bias and fairness, transparency and explainability, accountability, privacy and security, and ethical design, AI development company can lead the way in developing AI systems that are trustworthy, fair, and beneficial. As AI continues to evolve, ongoing commitment to ethical principles will be crucial in navigating the complex landscape of AI development and deployment.
2 notes
·
View notes
Photo

Against the Grain
The intelligent part of artificial intelligence (AI) is mimicking our thought processes – making decisions based on logic and knowledge, and perhaps learning new rules along the way to hone these choices. But as deep-learning models grow more complex, the logic driving them can be lost in the code. Pictured under a scanning electron microscope, spotting different varieties of pollen grains, is a fresh challenge for artificial intelligence. A team of computer scientists and bioengineers sort grains by their different chemical and shape (morphological) properties using a form of explainable AI (xAI) – in which the artificial ‘thought processes’ are transparent and easy to follow. Apart making an excellent testing ground for xAI methods, the team are able to compare different models for classifying pollen, which may ultimately improve how we estimate pollen counts, make forecasts and promote advice to millions of people suffering allergies worldwide.
Written by John Ankers
Image from work by Sanja Brdar and colleagues
BioSense Institute - Research Institute for Information Technologies in Biosystems, University of Novi Sad, Novi Sad, Serbia
Image originally published with a Creative Commons Attribution 4.0 International (CC BY 4.0)
Published in Scientific Reports, February 2023
You can also follow BPoD on Instagram, Twitter and Facebook
#science#biomedicine#artificial intelligence#pollen#allergy#pollen grain#electron microscopy#explainable AI#AI
12 notes
·
View notes
Text
The Fascinating Rivalry Between ChatGPT and Elon Musk's AI Grok

In the realm of artificial intelligence, the recent buzz is all about the rivalry between OpenAI's ChatGPT and Elon Musk's AI model Grok. This competition not only showcases the rapid advancements in AI but also opens a dialogue about the future of technology in our lives. Let's delve into the intricacies of this rivalry and what it means for the AI industry. The Genesis of Grok and Its Comparison with ChatGPT https://twitter.com/ChatGPTapp/status/1733569316245930442 Grok, developed under Musk's guidance, represents a new wave in AI technology. It stands in direct competition with OpenAI's ChatGPT, a platform renowned for its conversational abilities and diverse applications. What sets Grok apart is its integration with real-time data, particularly through social media platforms, unlike the basic version of ChatGPT, which relies on data only up to 2023. The Clash Over Common Responses The rivalry took an intriguing turn when ChatGPT highlighted a case where Grok responded to a prompt with an almost identical answer to that of ChatGPT, even mentioning OpenAI. This incident reignited discussions about whether Grok was trained on OpenAI's code, a claim Musk has consistently denied. In response, Musk suggested that the similarity in responses could be due to ChatGPT scraping data from Grok's platform for training purposes. This allegation adds another layer of complexity to the ongoing debate about data usage and intellectual property in the AI domain. Musk's Engagement with Grok's Feedback https://twitter.com/linasbeliunas/status/1733547217649127598 Musk's active involvement in Grok's development and his response to public feedback, both positive and negative, is noteworthy. He has been seen reacting to user comments about Grok on social media, sometimes with humor, indicating his serious investment in the platform's success. https://aieventx.com/elon-musk-pits-chat-gpt-against-grok-in-an-ai-duel-over-the-trolley-problem/ Differing Functionalities and Access to Information A significant difference between ChatGPT and Grok lies in their access to information. While ChatGPT requires a subscription to access real-time data, Grok reportedly has this feature inherently, thanks to its integration with social media data. The Controversy and Clarifications The controversy regarding Grok's data sources led to a statement from Igor Babuschkin, an X user affiliated with xAI. He explained that the similarities in responses might have occurred because Grok inadvertently picked up ChatGPT outputs while being trained on a vast array of web data. He reassured that no OpenAI code was used in Grok's development, emphasizing the rarity of such incidents and promising rectifications in future versions. Broader Implications in the AI Race This rivalry between ChatGPT and Grok exemplifies the broader competition in the AI industry, where big tech companies vie to outperform each other. It raises questions about the ethics of AI development, data privacy, and the potential for AI models to inadvertently overlap in their learning processes. The Future of AI: OpenAI and Musk's Vision As AI continues to evolve, the direction taken by platforms like ChatGPT and Grok will significantly influence the industry's trajectory. Musk's vision for Grok and OpenAI's ongoing innovations with ChatGPT are shaping a future where AI is not only more integrated into our daily lives but also more contentious in terms of its development and application. Conclusion The rivalry between ChatGPT and Grok is more than a technological competition; it is a reflection of the dynamic and sometimes tumultuous nature of AI evolution. As these platforms continue to grow and adapt, they will undoubtedly shape the landscape of AI, posing new challenges and opening up unprecedented possibilities in the realm of artificial intelligence.
Read the full article
3 notes
·
View notes
Text
Exploring the Next Frontier of Data Science: Trends and Innovations Shaping the Future
Data science has evolved rapidly over the past decade, transforming industries and reshaping how we interact with data. As the field continues to grow, new trends and innovations are emerging that promise to further revolutionize how data is used to make decisions, drive business growth, and solve global challenges. In this blog, we will explore the future of data science and discuss the key trends and technologies that are likely to shape its trajectory in the coming years.
Key Trends in the Future of Data science:
Artificial Intelligence (AI) and Machine Learning Advancements The integration of artificial intelligence (AI) and machine learning (ML) with data science is one of the most significant trends shaping the future of the field. AI algorithms are becoming more sophisticated, capable of automating complex tasks and improving decision-making in real-time. Machine learning models are expected to become more interpretable and transparent, allowing businesses and researchers to better understand how predictions are made. As AI continues to improve, its role in automating data analysis, pattern recognition, and decision-making will become even more profound, enabling more intelligent systems and solutions across industries.
Explainable AI (XAI) As machine learning models become more complex, understanding how these models make decisions has become a crucial issue. Explainable AI (XAI) refers to the development of models that can provide human-understandable explanations for their decisions and predictions. This is particularly important in fields like healthcare, finance, and law, where decision transparency is critical. The future of data science will likely see a rise in demand for explainable AI, which will help increase trust in automated systems and enable better collaboration between humans and machines.
Edge Computing and Data Processing Edge computing is poised to change how data is processed and analyzed in real-time. With the rise of the Internet of Things (IoT) devices and the increasing volume of data being generated at the "edge" of networks (e.g., on smartphones, wearables, and sensors), there is a growing need to process data closer to where it is collected, rather than sending it to centralized cloud servers. Edge computing enables faster data processing, reduced latency, and more efficient use of bandwidth. In the future, data science will increasingly rely on edge computing for applications like autonomous vehicles, smart cities, and real-time healthcare monitoring.
Automated Machine Learning (AutoML) Automated Machine Learning (AutoML) is another trend that will shape the future of data science. AutoML tools are designed to automate the process of building machine learning models, making it easier for non-experts to develop predictive models without needing deep technical knowledge. These tools automate tasks such as feature selection, hyperparameter tuning, and model evaluation, significantly reducing the time and expertise required to build machine learning models. As AutoML tools become more advanced, they will democratize access to data science and enable more organizations to leverage the power of machine learning.
Data Privacy and Security Enhancements As data collection and analysis grow, so do concerns over privacy and security. Data breaches and misuse of personal information have raised significant concerns, especially with regulations like GDPR and CCPA in place. In the future, data science will focus more on privacy-preserving techniques such as federated learning, differential privacy, and homomorphic encryption. These technologies allow data models to be trained and analyzed without exposing sensitive information, ensuring that data privacy is maintained while still leveraging the power of data for analysis and decision-making.
Quantum Computing and Data Science Quantum computing, while still in its early stages, has the potential to revolutionize data science. Quantum computers can perform calculations that would be impossible or take an impractically long time on classical computers. For example, quantum algorithms could dramatically accelerate the processing of complex datasets, optimize machine learning models, and solve problems that currently require huge amounts of computational power. Although quantum computing is still in its infancy, it holds promise for making significant strides in fields like drug discovery, financial modeling, and cryptography, all of which rely heavily on data science.
Emerging Technologies Impacting Data Science:
Natural Language Processing (NLP) and Language Models Natural Language Processing (NLP) has made huge strides in recent years, with language models like GPT-4 and BERT showing remarkable capabilities in understanding and generating human language. In the future, NLP will become even more integral to data science, allowing machines to understand and process unstructured data (like text, speech, and images) more effectively. Applications of NLP include sentiment analysis, chatbots, customer service automation, and language translation. As these technologies advance, businesses will be able to gain deeper insights from textual data, enabling more personalized customer experiences and improved decision-making.
Data Democratization and Citizen Data Science Data democratization refers to the movement toward making data and data analysis tools accessible to a broader range of people, not just data scientists. In the future, more organizations will embrace citizen data science, where employees with little to no formal data science training can use tools to analyze data and generate insights. This trend is driven by the growing availability of user-friendly tools, AutoML platforms, and self-service BI dashboards. The future of data science will see a shift toward empowering more people to participate in data-driven decision-making, thus accelerating innovation and making data science more inclusive.
Augmented Analytics Augmented analytics involves the use of AI, machine learning, and natural language processing to enhance data analysis and decision-making processes. It helps automate data preparation, analysis, and reporting, allowing businesses to generate insights more quickly and accurately. In the future, augmented analytics will play a major role in improving business intelligence platforms, making them smarter and more efficient. By using augmented analytics, organizations will be able to harness the full potential of their data, uncover hidden patterns, and make better, more informed decisions.
Challenges to Address in the Future of Data science:
Bias in Data and Algorithms One of the most significant challenges that data science will face in the future is ensuring fairness and mitigating bias in data and algorithms. Data models are often trained on historical data, which can carry forward past biases, leading to unfair or discriminatory outcomes. As data science continues to grow, it will be crucial to develop methods to identify and address bias in data and algorithms to ensure that machine learning models make fair and ethical decisions.
Ethical Use of Data With the increasing amount of personal and sensitive data being collected, ethical considerations in data science will become even more important. Questions around data ownership, consent, and transparency will need to be addressed to ensure that data is used responsibly. As the field of data science evolves, there will be a growing emphasis on establishing clear ethical guidelines and frameworks to guide the responsible use of data.
Conclusion: The future of Data science is full of exciting possibilities, with advancements in AI, machine learning, quantum computing, and other emerging technologies set to reshape the landscape. As data science continues to evolve, it will play an even more central role in solving complex global challenges, driving business innovation, and enhancing our daily lives. While challenges such as data privacy, ethical concerns, and bias remain, the future of data science holds enormous potential for positive change. Organizations and individuals who embrace these innovations will be well-positioned to thrive in an increasingly data-driven world.
0 notes
Text
Unlocking Transparency in Healthcare Decision Making with Explainable AI
Introduction Implementing Explainable AI (XAI) for decision making in healthcare is a crucial step towards increasing trust in AI-driven medical decisions. XAI provides insights into how AI models arrive at their conclusions, enabling healthcare professionals to understand and validate the decisions made by AI systems. This article will guide readers through the process of implementing XAI for…
0 notes
Text
Elon Musk's xAI: Advancing AI for Universal Understanding

In the ever-evolving landscape of artificial intelligence, Elon Musk, a figure synonymous with technological disruption, has once again captured global attention with the launch of his latest venture: xAI. This ambitious project aims to redefine AI's role in fostering universal understanding and solving humanity's greatest challenges.
What is xAI?
xAI, short for "Explainable Artificial Intelligence," is Elon Musk's bold foray into developing cutting-edge AI systems designed to benefit humanity. The initiative stands apart from traditional AI projects by focusing not just on the capabilities of AI but on its transparency and alignment with human values. Musk’s mission is clear: create AI models that are not only powerful but also interpretable and universally accessible.
Launched in mid-2023, xAI operates with a central ethos of fostering cooperation between AI and humans. This distinguishes it from other AI ventures that often prioritize raw computational power or niche applications.
Aims and Objectives of xAI
Musk’s vision for xAI aligns closely with his long-standing concerns about AI safety and the potential risks posed by unchecked AI development. The project's primary objectives include:
Building Safe AI Models: By focusing on explainability, xAI seeks to ensure AI systems make understandable and justifiable decisions, reducing the risks of unintended consequences.
Fostering Universal Understanding: xAI aims to bridge the gap between diverse human perspectives by developing AI capable of synthesizing and interpreting vast amounts of data in a contextually relevant and neutral manner.
Tackling Global Challenges: From climate change to resource allocation, xAI aspires to apply its technologies to pressing global issues, offering innovative solutions through intelligent systems.
Democratizing AI: Musk has often emphasized the importance of making AI tools accessible to all. xAI is expected to produce platforms that empower individuals and organizations globally, regardless of their technical expertise.
How xAI Differs from Other Musk Ventures
While Musk’s other ventures, such as Tesla, SpaceX, and Neuralink, focus on transforming transportation, space exploration, and neural enhancement, xAI zeroes in on the cognitive potential of AI. It also contrasts with OpenAI, which Musk co-founded but later distanced himself from due to concerns over its trajectory.
xAI is unique in its emphasis on explainability, a crucial factor in ensuring that AI systems remain aligned with human intent. This focus positions xAI as a leader in ethical AI development.
The Role of Explainable AI (XAI)
Explainable AI is a burgeoning field within AI research that addresses one of the technology's most significant criticisms: opacity. Often described as "black boxes," advanced AI systems can produce results without revealing the underlying reasoning.
xAI’s commitment to explainability ensures that its models provide clear insights into their decision-making processes. This approach is particularly important for:
High-stakes applications, such as healthcare, where trust and transparency are critical.
Regulatory compliance, ensuring AI adheres to legal and ethical standards.
Public trust, addressing fears of AI systems behaving unpredictably.
Challenges Ahead for xAI
As with any groundbreaking endeavor, xAI faces several challenges:
Technical Complexity: Developing explainable systems without compromising performance is a significant technical hurdle.
Ethical Dilemmas: Aligning AI with universally accepted values is a daunting task, given the diversity of human cultures and perspectives.
Competition: xAI enters a crowded field dominated by tech giants like Google, Microsoft, and Meta, which are also investing heavily in AI research.
The Future of xAI and AI at Large
Elon Musk envisions xAI as a cornerstone in humanity's journey toward AI-powered enlightenment. By focusing on safety, transparency, and universal accessibility, xAI has the potential to revolutionize how humans interact with intelligent systems.
In the coming years, xAI could play a pivotal role in fostering collaboration between AI and humans, solving complex problems, and enhancing global understanding. While the road ahead is undoubtedly challenging, Musk’s track record of turning ambitious ideas into reality suggests that xAI could very well become a defining force in the next phase of AI evolution.
As xAI continues to develop, it raises a profound question for humanity: how can we ensure that the AI we create reflects the best of our shared values? Elon Musk and his team at xAI may soon provide an answer, shaping not just the future of AI but the future of humanity itself.
0 notes
Text
The Convergence of Databases and Artificial Intelligence: Revolutionizing Application Development
The advent of sophisticated chatbots and Large Language Models (LLMs) has marked a pivotal moment in the evolution of data management and application development. These Artificial Intelligence (AI) powerhouses generate unprecedented volumes of unstructured data and intricate query patterns, exposing the limitations of traditional database architectures. In response, the tech industry is witnessing a groundbreaking convergence of database management and AI, poised to transform the application development landscape.
At the heart of this revolution lies the challenge of accommodating the vast, complex data sets produced by AI models. Conventional databases, designed to handle structured data and predictable queries, struggle to maintain efficiency and resilience in the face of such demands. To bridge this gap, innovators are developing novel database solutions that integrate seamlessly with AI-driven applications. A key aspect of this approach involves supporting advanced query methodologies, such as Retrieval Augmented Generation (RAG) and semantic search, which significantly enhance database performance and scalability.
The integration of AI-centric extensions into database management systems represents a paradigm shift in application development. By embedding LLMs directly into databases, developers can achieve transformative leaps in scalability. Benchmarks from high-traffic datasets have shown promising results, with response times under 2 seconds even at peak loads. This feat is made possible by dynamic resource allocation, which intelligently scales resources based on query demands, mitigating the increased computational requirements typically associated with AI adoption. Furthermore, the implementation of comprehensive end-to-end encryption ensures the safeguarding of sensitive information, a critical consideration in the context of AI-driven queries.
This convergence of databases and AI is set to profoundly impact the application development process. By streamlining the integration of multiple tools and leveraging open-source solutions, developers can anticipate a simplified development environment. Enhanced accessibility will likely democratize access to AI-driven technologies, fostering innovation across a broader spectrum of industries. Ultimately, the evolution of databases into active, AI-driven entities within the technological ecosystem will irreversibly alter the landscape of application development.
However, this rapid evolution also presents challenges, particularly in managing diverse contributor inputs in open-source projects and ensuring the stability and security of AI-infused databases. To navigate these complexities, a dual approach is emerging: the implementation of rigorous testing frameworks to guarantee project integrity, alongside community governance models that balance collaborative innovation with the need for oversight.
As the technological horizon continues to unfold, the integration of Explainable AI (XAI) capabilities into next-generation databases is expected to play a pivotal role. By providing unparalleled transparency into AI-driven query decisions, XAI will not only enhance trust in these systems but also facilitate deeper insights into their operational dynamics. Moreover, the ongoing engagement with ethical AI discourses underscores a growing recognition of the need to harmonize the pursuit of innovation with the paramount responsibility of safeguarding user trust and privacy in the deployment of LLMs within databases.
The convergence of databases and Artificial Intelligence heralds a revolutionary era in application development, marked by enhanced scalability, simplified development processes, and redefined database management paradigms. As this technological symbiosis continues to evolve, it is clear that the future of data-driven innovation will be shaped by the thoughtful integration of AI into the very fabric of database architectures.
Avthar Sewrathan: How to Build Smarter AI Applications with PostgreSQL (Craig Smith, Eye on AI, November 2024)
youtube
Tuesday, November 19, 2024
#database innovation#artificial intelligence integration#application development#technological convergence#ai-centric solutions#interview#ai assisted writing#machine art#Youtube
0 notes
Text
The Future of AI Agent Development: Trends and Innovations
Artificial Intelligence (AI) agents are transforming industries by automating processes, enhancing decision-making, and providing personalized user experiences. As AI continues to advance, the development of intelligent agents is becoming a cornerstone of technological innovation.
In this blog, we explore the future of AI agent development, highlighting emerging trends, groundbreaking innovations, and the potential impact on society.
What Are AI Agents?
AI agents are software programs designed to perform tasks autonomously, often leveraging machine learning (ML), natural language processing (NLP), and other AI technologies. They can range from simple chatbots to complex systems capable of autonomous decision-making in dynamic environments.
Emerging Trends in AI Agent Development
1. Multimodal AI
Multimodal AI agents process and interpret multiple forms of data, such as text, images, audio, and video, to make more informed decisions. For example, healthcare AI agents that combine patient records with diagnostic images can provide more accurate assessments.
2. Context-Aware Intelligence
Future AI agents will become more context-aware, understanding not just user inputs but the environment and situation. This capability will enable agents to offer highly personalized and situationally appropriate responses, essential for fields like smart home automation and autonomous vehicles.
3. Low-Code and No-Code Development
Low-code and no-code platforms are democratizing AI agent development, enabling non-technical users to create sophisticated agents. These platforms reduce the dependency on extensive coding skills, fostering innovation across various industries.
4. Federated Learning for Decentralized AI
Federated learning is transforming how AI agents are trained. Instead of relying on centralized data, agents can learn locally from user data while maintaining privacy. This approach is critical for applications like personalized healthcare and financial advisory.
5. Explainable AI (XAI)
As AI agents make increasingly complex decisions, understanding their reasoning becomes crucial. Explainable AI ensures transparency by allowing developers and users to comprehend and trust the decisions made by AI agents.
6. Integration with IoT
The Internet of Things (IoT) is a key driver in the evolution of AI agents. Agents embedded in IoT devices will provide seamless interactions, whether in smart homes, industrial automation, or healthcare devices.
Innovations Driving the Future of AI Agents
1. Neuro-Symbolic AI
Combining the strengths of symbolic reasoning and neural networks, neuro-symbolic AI offers a hybrid approach to problem-solving. This innovation enhances an AI agent’s ability to handle both logical reasoning and unstructured data.
2. Generative AI for Customization
Generative AI models like GPT are enabling the creation of highly personalized AI agents. These agents can craft unique outputs, such as personalized responses in customer service or tailored educational content.
3. Edge AI for Real-Time Processing
Edge AI allows agents to process data locally on devices rather than relying on cloud infrastructure. This innovation reduces latency, enhances privacy, and ensures faster response times in applications like autonomous drones and wearable technology.
4. Advanced Reinforcement Learning
Reinforcement learning is empowering AI agents to learn through interaction and feedback. Advanced techniques like multi-agent reinforcement learning (MARL) enable collaboration and competition among multiple agents, useful in robotics and game AI.
5. Human-Agent Collaboration
The focus is shifting towards AI agents that complement human abilities rather than replace them. For example, AI-powered decision support systems in industries like law and medicine act as co-pilots for professionals.
Impact on Industries
Healthcare
AI agents will play a vital role in diagnostics, remote patient monitoring, and personalized treatment plans. Innovations in NLP and computer vision are already improving early disease detection.
Finance
From fraud detection to algorithmic trading, AI agents are enhancing security and efficiency in financial systems. Personal finance agents are also becoming trusted advisors for budgeting and investment.
Education
AI-powered tutors and personalized learning platforms are revolutionizing education, adapting to each student’s pace and style of learning.
Retail
Virtual shopping assistants and AI-driven inventory management systems are transforming how businesses interact with customers and manage operations.
Transportation
Autonomous driving agents are a major focus in transportation, with advancements in real-time decision-making and object recognition pushing the boundaries of safety and efficiency.
Challenges and Ethical Considerations
While the future of AI agent development is promising, challenges persist:
Data Privacy: Ensuring agents respect user privacy while leveraging personal data.
Bias in AI: Developing fair and unbiased agents that don’t perpetuate stereotypes.
Job Displacement: Balancing automation with the need for human jobs and social equity.
Security: Preventing malicious use of AI agents in cyberattacks or misinformation campaigns.
Conclusion
The future of AI agent development is brimming with potential, driven by innovative technologies and trends that promise to reshape industries and daily life. As developers and stakeholders, it’s imperative to focus on creating ethical, transparent, and user-centric AI agents that augment human capabilities and foster societal growth.
AI agents are not just tools of convenience—they are becoming indispensable partners in our journey toward a smarter and more connected world.
0 notes